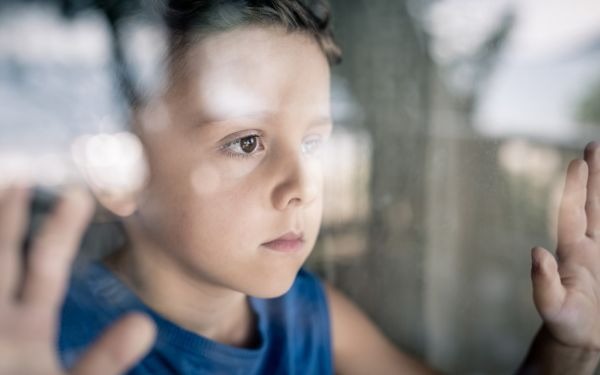
by Emily Keddell and Tony Stanley
Predictive algorithms promoted and used by Hackney council to identify those most in need of scarce preventive services is an important issue for us to think more deeply about.
In that article, Steve Liddicott reasoned that in the context of reduced spending on prevention services, identifying those most in need earlier could prevent children ending up in more intensive services like child protection. The article presents the reduction of spending as a given and promotes algorithms as a method of distributing what little is left. In this response, we argue this is not a benign activity and is ethically fraught.
Firstly, the reduction of services leaves significant holes that an algorithm can’t fix. It reduces the problem to one of accurate fit, rather than inadequate size. It also diverts more funds away from the provision of services into expensive software development and maintenance that could be spent on services themselves. How much did this all cost?
The use of algorithmic and other statistical methods in preventive service distribution and child protection decision-making is entangled with ethical and practical data issues. The source and quality of the predictive variables, the quality of data linkage, the type of statistical methods used, the outcome the algorithm is trained on and the accuracy of the algorithm all require examination. Ethical issues are numerous – the lack of explicit consent to use people’s data in this way is of concern to us.
Assumptions
Stigma caused by children identified to be ‘at risk’ is likely to follow. As Cathy O’Neil writes, algorithms are the expression of the assumptions of those who construct them. What outcomes are considered worthy of prediction, for example, is one such assumption.
Substantiation of concern or removal of the child decisions are often the outcome algorithms are trained on, but these are human decisions subject to the same biases and variability that algorithms claim to reduce. What happens to children not substantiated is unknown. This means that the algorithm can’t ‘learn’ accurate rules properly as they don’t have the range of data needed to do so.
Few have access to incidence studies, and reliance on child protection or other administrative data conflates true incidence of need, harm and abuse with just contact with child welfare systems.
The big problem in an algorithm drawing on administrative data is that it will contain bias relating to poverty and deprivation. Where council housing data is used, for example, those who don’t need council housing will be absent. Those caught up in criminal justice systems and social services of any kind lead to an oversampling of the poor.
Big datasets such as these make some people invisible, while others become super visible, caught in the glare of the many data points that the council or government holds about them. Where such processes occur under the veil of commercial sensitivity, even the most basic of ethical or data checks are difficult to undertake.
Maverick practices
The problem with private companies partnering with public bodies, as in a recent Chicago case, is that the weighting of variables and identification of outcomes that the algorithm is trying to predict are beyond the reach of transparent inspection – it happens in a ‘black-box’ that we can’t open. We can’t check. We can’t see what is happening.
This can lead to unregulated maverick practices and contributes to our inability to offer people identified by algorithms with the ‘right to an explanation’ about decisions made about them recently written into the European General Data Protection Regulation.
How do social workers or family support workers explain to the family that the phone call or knock on the door resulted from today’s routine computations? Is this helping those that may need our help? At least the use of algorithmic prediction to determine who to offer community and preventative services to is relatively benign, as they are voluntary. What happens when the family refuses or declines the offer of early help? Does this add another risk factor to the predictive model? Is the algorithm machinery then producing a revised higher risk definition? Probably.
There are numerous examples of this in the US, recently highlighted in Chicago with the Eckerd tool, and the Alleghney Family Safety tool currently in use or in New Zealand where a recent trial at intake used a similar model to identify those deemed high risk.
Accuracy
Apart from the bias inherent in the data used, the actual accuracy of such tools is fairly limited. One tool developed in New Zealand was just 25% accurate at the top decile of risk over five years – meaning 75% of those identified by the tool as high risk had no findings. While the claim is often made that human decision-makers are inaccurate and biased, where an algorithm identifies someone as high risk, the possibility of false positives are high, and the conflict between a probability based on collective risk, and the human rights concept that protects the rights of individuals is stark.
An individual cannot be held responsible for the statistical likelihood of belonging to a collective of those who are similar to them in one, ten or a hundred ways.
Legal responsibility and fairness relies on individual differentiation. So while human decision makers are certainly far from perfect, ascertaining culpability or need based on algorithms should be approached with caution.
Virginia Eubanks’ recent book, Automating Inequality: How High-Tech Tools Profile, Police, and Punish the Poor, claims the use of such methods recreates the ‘poorhouse’ of old, by cloaking both the structures that lead to poverty, and the implicit beliefs about the poor in a veneer of scientific legitimacy.
They then ensnare some people in an inescapable web of data generated by accidents of birth that they cannot escape from. Technological advances in data systems are not our concern here, rather we are concerned with arguments that algorithm usage is a benign activity to help those in need. It most certainly is not.
Emily Keddell is a senior lecturer in social work at Otago university. Tony Stanley is a social worker , most recently employed as chief social worker for Birmingham children’s services, and has recently moved to New Zealand.
In conjunction with other social worker assessment tools is fine. Alone and without consideration of other assessment tools algorithms universally I think is not a single line use tool to rely upon.
I guess the main issue, as stated in the article, is that RPM tools like this bring up particular populations – poor, of colour, unemployed – over others. So those families are going to be interviewed or investigated. They’re going to have more information fed into the system, and probably will become more heavily surveilled in turn. If a family comes up as high risk on a pre-assessment, even for structural reasons they can’t control, you can’t ignore that (what if it’s right?). I think the main issue with these tools is the politico-economic environment they’re created/used in. Ofc no risk assessment is free from this, but it being reduced to an algorithm that is controlled centrally without any human input is pretty dangerous.
Eboni: Even when predictive models are explicitly intended to be used to inform (not supplant) caseworker decision-making, many defer to the tool’s apparent objectivity, and supervisors train to the tool. In Allegheny County, I asked a manager what she did when the algorithmic score and the intake workers’ evaluations diverged. She said it was an opportunity to teach caseworkers what they are doing wrong.
Where do they come up with this stuff?
Helpful piece that explores the tensions with a i – l
I don’t think there are any more or less ethical issues both are person designed made/involve persons.
The real question is whether the artificial intelligence(which is person made) is any better or better than the ‘normal /live’ person based intelligence at the task in hand, improving the welfare/safeguarding services and outcomes
I agree with the authors of he piece ( and thank you for writing it ) ‘big brother’ algorithms are extremely problematic ethically. There is on the other hand, a place for big data – See
https://www.childrenscommissioner.gov.uk/wp-content/uploads/2018/03/Childrens-Commissioners-Business-Plan-2018-19.pdf
The Children’s Commissioner is a believer in it to analyse LAs and CCG’s spending patterns as just two examples.
She also raises serious concerns about big tech collecting, mining and selling children’s information. I’m not really clear on why it is OK if a LA does the same. In some ways it is even more sinister.
Thanks again for the very good piece.
I think predictive algorithms could become the stop and search of child protection services. And we know how that works out for police and community relations.